Real-time probabilistic flood inundation mapping is crucial for flood risk warning and decision-making dur-ing the emergency period before an upcoming flood event. Considering the high uncertainties involved in the modeling of a nonlinear and complex flood event, providing a deterministic flood inundation map can be erroneous and mis- leading for reliable and timely decision-making. The conven-tional flood hazard maps provided for different return periods cannot also represent the actual dynamics of flooding rivers. Therefore, a real-time modeling framework that forecasts the inundation areas before the onset of an upcoming flood is
of paramount importance. Sequential data assimilation (DA) techniques are well known for real-time operation of physical models while accounting for existing uncertainties. In this study, we present a DA hydrodynamic modeling framework where multiple gauge observations are integrated into the LISFLOODFP model to improve its performance. This study utilizes the ensemble Kalman filter (EnKF) in a mul- tivariate fashion for dual estimation of model state variables and parameters where the correlations among point source observations are taken into account… read more
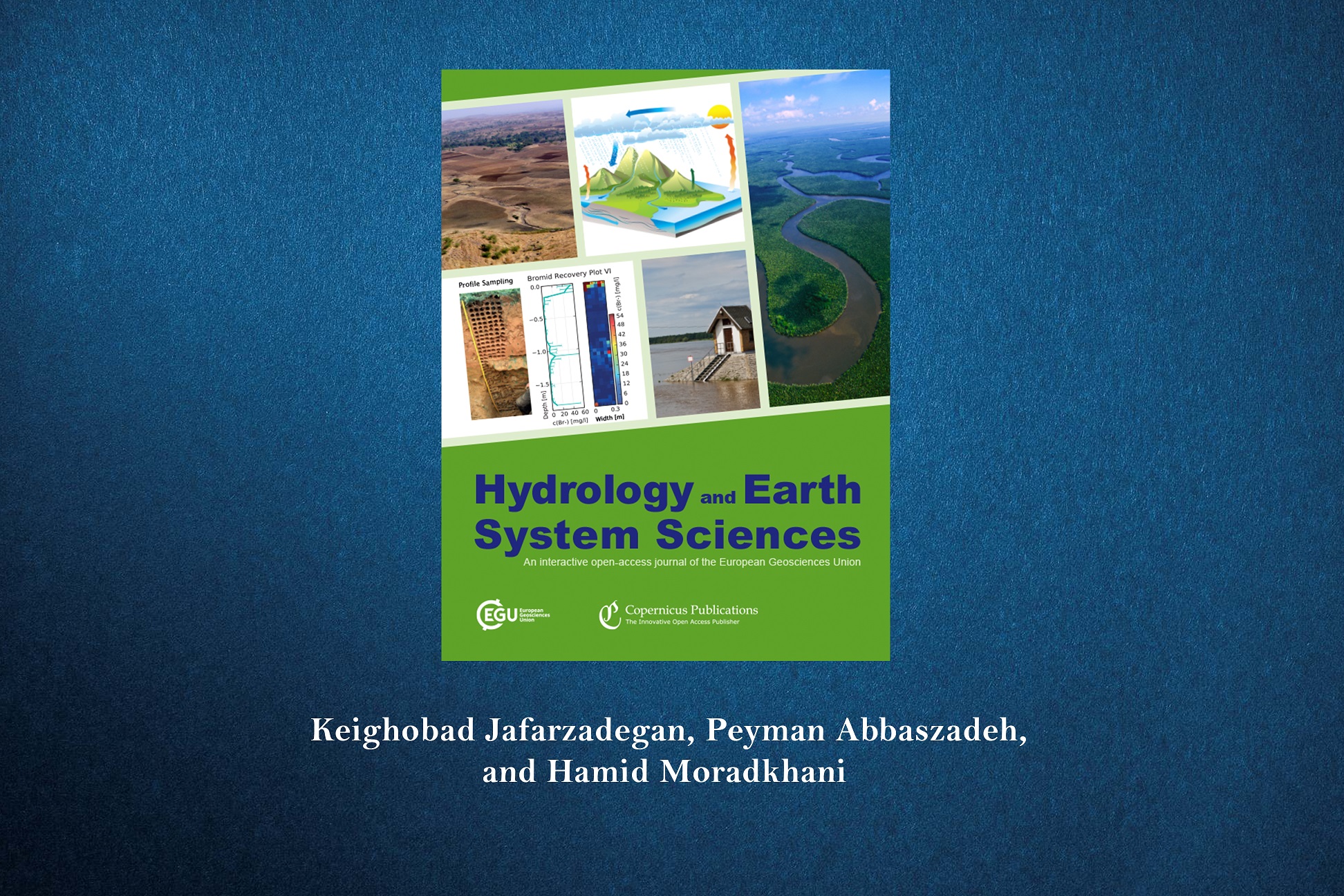
KJNPAPER
No comment